Get ready to explore the exciting world of autonomous vehicles and the future of AI. This article will explore computer vision’s challenges in autonomous vehicles and how it shapes our driving experiences. From variability in environmental conditions to object detection complexities, computer vision encounters hurdles.
We’ll also touch upon the vital role of data labeling and annotation services in training AI models. So, fasten your seatbelts for a thrilling journey into the future of AI in autonomous vehicles.
Understanding Computer Vision
Let’s understand what computer vision is all about. It’s like giving eyes to machines, allowing them to “see” and interpret their surroundings. For autonomous vehicles, computer vision is the technology that enables them to understand the world around them, detect objects, recognize road signs, and make decisions based on what they “see.”
Challenges in Computer Vision for Autonomous Vehicles
Achieving accurate computer vision in autonomous vehicles isn’t a walk in the park. Several challenges need to be tackled. From the variability in environmental conditions and lighting to the complexities of object detection and recognition, there’s a lot for AI to overcome.
That’s where image annotation services come into play. These services provide labeled data, training the computer vision algorithms to recognize and interpret various objects and scenarios. Image annotation involves marking objects of interest in images, such as pedestrians, vehicles, traffic signs, and road boundaries. It’s a crucial step in training AI models for autonomous vehicles, as it helps them learn and improve their understanding of the world.
Variability in Environmental Conditions and Lighting
The variability in environmental conditions is one of the major challenges computer vision faces in autonomous vehicles. Adverse weather conditions like rain, snow, or fog can significantly impact the performance of computer vision systems. According to a study conducted by the University of Michigan Transportation Research Institute, autonomous vehicles experience a 40% increase in detection errors during rain or snowfall.
Low-light and high-glare situations also pose challenges to computer vision. Accurately detecting objects and interpreting their surroundings under varying lighting conditions is crucial for safe autonomous driving. Innovative algorithms and sensor technologies are being developed to address these challenges, ensuring that autonomous vehicles can “see” clearly regardless of the lighting conditions.
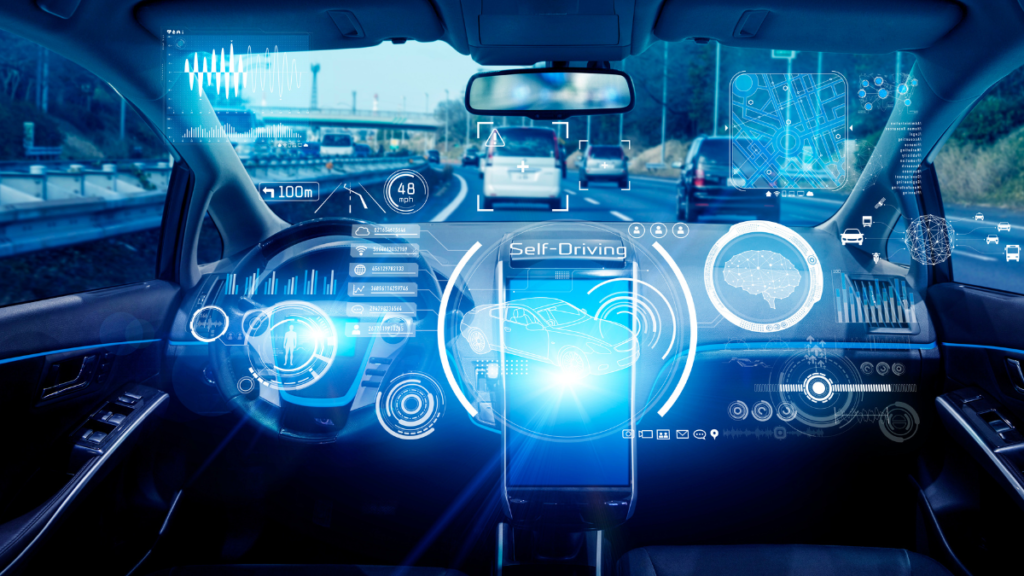
Object Detection and Recognition
Another significant challenge is object detection and recognition. Identifying and classifying objects in real time is complex, especially in crowded and dynamic traffic scenarios. The AI models need to accurately distinguish between pedestrians, cyclists, vehicles, and other objects to make informed decisions on the road. Overcoming challenges like occlusions, where objects may be partially hidden from view, requires sophisticated algorithms and extensive training with annotated data. That’s why hiring reliable data annotation services like oworkers helps a lot in addressing computer vision challenges in autonomous vehicles.
Motion Prediction and Tracking
Motion prediction and tracking are also crucial aspects of computer vision in autonomous vehicles. Predicting the future paths of moving objects and tracking them accurately is vital for safe navigation. This includes anticipating the behavior of pedestrians, cyclists, and other vehicles to make timely decisions and avoid collisions. It’s an ongoing area of research and development to enhance the capabilities of computer vision systems in real-time, dynamic traffic environments.
Overcoming the Challenges
Advances in Deep Learning and Neural Networks
Deep learning and neural network advancements have revolutionized computer vision in autonomous vehicles. Deep learning algorithms can learn complex patterns and features from vast data, improving object detection and recognition accuracy. Training these models requires large-scale datasets with annotated images, where image annotation services play a crucial role.
Sensor Fusion for Enhanced Perception
Sensor fusion is another approach to enhance the perception capabilities of autonomous vehicles. Combining data from multiple sensors, such as cameras, radar, and LiDAR (Light Detection and Ranging), allows to understand the environment more efficiently. Each sensor provides unique information, and integrating their inputs gives the AI system a more reliable perception of its surroundings.
Continuous Learning and Adaptation
Continuous learning and adaptation are essential for computer vision in autonomous vehicles. The models need to constantly update and refine their understanding of the world to adapt to new scenarios and changing road conditions. Real-time data from sensors, feedback loops, and algorithm updates help the AI system improve its performance over time.
The Future of AI in Autonomous Vehicles
The future of AI in autonomous vehicles is promising. Advancements in computer vision technology are paving the way for more sophisticated algorithms and architectures. Researchers and engineers are exploring new sensing technologies, such as advanced cameras and improved LiDAR systems, to enhance perception capabilities.
The impact of AI in autonomous vehicles goes beyond convenience and efficiency. According to the World Health Organization, road traffic accidents claim over 1.35 million lives each year globally. Autonomous vehicles have are capable to reduce accidents by eliminating human error, which is responsible for the majority of crashes. Additionally, they can improve traffic flow, reduce congestion, and provide accessible transportation options for people with limited mobility.
However, as with any emerging technology, ethical considerations and societal impacts must be addressed. Privacy, security, and algorithmic biases are important discussions surrounding autonomous vehicles and AI. Striking a balance between human control and autonomy in decision-making is crucial to ensure safety and trust in these systems.